The research group “Hydrolab” coordinated by Prof. Salvatore Manfreda participates in the COST project “Harmonious – Harmonisation of UAS techniques for agricultural and natural ecosystems monitoring” with research teams from important European universities (Manfreda et al., 2018). The research activities related to the WG4 “River morphology and streamflow monitoring” aim to provide a scientific contribution to the definition of guidelines for river monitoring using Unmanned Aerial Systems (UASs). The research activities are addressed to the identification of methodologies and procedures to optimise surface flow velocities estimation by field experiences and numerical modelling. Specifically, the research activities concern the analysis of surface velocity field from optical sensors mounted on mobile platforms (UAS).
The research contributions involve the following topics: 1) the construction of a database of case studies with supporting benchmark measurements; 2) the experimentation of image pre-processing techniques to optimise surface velocity estimates; 3-4) the application and comparison of image velocimetry techniques and tools to real case studies; and, 5) the elaboration of guidelines for hydrological monitoring by UAS.

The Hydrolab research group in the last years has actively contributed to:
(1) the construction of a database of case studies conducted in different river systems of the Basilicata region using optical systems from UAS and current meter techniques for surface velocity estimations. These field experiences are part of the video collection published by Perks et al. (2020), containing 13 case studies conducted in 6 different countries in the world. The database contains pre-processed frames and reference measurements.
(2a) the implementation and comparison of image stabilisation techniques aimed at removing the effects of camera movements induced by wind, vibrations or operator inexperience (http://10.5194/hess-2021-112). VISION software is an open-source tool written in Matlab for video stabilisation using the automatic detection of key features without direct operator control. Code is available at the following web address: http://10.17605/OSF.IO/HBRF2.
(2b) the identification and quantification of spatial and temporal characteristics of seeding during the acquisition time to maximise the performance of image processing techniques. For this purpose, three metrics have been introduced based on the calculation of the (i) seeding density, (ii) index of dispersion of tracers, and (iii) coefficient of variation of tracer dimension. These metrics have statistical significance on image-based performances as reported in Dal Sasso et al. (2020).
Simultaneously, the generation of synthetic images reproducing some river conditions allowed to quantify the error associated with the Particle Tracking Velocimetry (PTV) and Large Scale Particle Image Velocimetry (LSPIV). Using this framework, Pizarro et al. (2020) introduced the Seeding Distribution Index (SDI) as a parameter that synthesises the seeding conditions in the field, merging seeding and spatial distribution characteristics. This dimensionless index was tested in some field case studies for describing the heterogeneous spatial distribution of tracers and the tendency to form clusters.
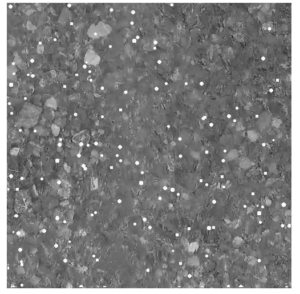
The synthetic images and the calculation codes relating to the metrics and the SDI index are published at the following link: https://doi.org/10.17605/OSF.IO/8EGQW.
The SDI index combined with a novel methodology of video acquisitions can allow to identify the best footage to analyse limiting processing times. This procedure has been successfully applied in several case studies and at various spatial scales (cross-section and sub-sectors), improving optical image processing techniques by 20-50%. These findings are reported in the works of Pizarro et al. (2020) and Dal Sasso et al. (2021). Code relating to this methodology is available at the following link: 48310.17605/OSF.IO/3AJNR.
(3-4) A comparison between image processing techniques and software on a real case study is reported in Pearce et al. (2020). In this work, a sensitivity analysis of the characteristic parameters of the individual techniques is carried out. Regarding the PTV technique, the results obtained in the work of Dal Sasso et al. (2018), regarding the identification of the optimal setting using synthetic images, have been used for this purpose.
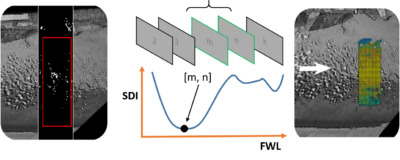
(5) The activities carried out and in progress are aimed to draw up the guidelines for hydrological monitoring From UAS. The chapter of Manfreda et al. (2019) provides the first contribution in this direction “New Insights Offered by UAS for River Monitoring” (10.1201/9780429244117-10) contained in the book “Applications of Small Unmanned Aircraft Systems” edited by Sharma.