Floods are one of the most widespread and devastating natural disasters worldwide. Understanding flood risk is paramount for managing the far-reaching socio-economic and environmental impacts of these events.
Researchers have tirelessly worked to develop innovative, data-driven solutions for tackling this challenge. Among these, hydrogeomorphic methods have emerged as a powerful tool by leveraging historical flood data and digital elevation models (DEMs). For detailed insights, explore the works of Tavares da Costa et al. (2019), Annis et al. (2019), Nardi et al. (2006, 2013, 2019), Clubb et al. (2017), Jafarzadegan and Merwade (2017), De Risi et al. (2015), and Degiorgis et al. (2012).
The journey to understanding flood-prone areas began with Kirkby (1975), who introduced the TOPMODEL topographic index. This index has since proven itself as a reliable indicator of flood-prone regions (Manfreda et al., 2011; De Risi et al., 2013; Jalayer et al., 2014). Manfreda et al. (2011) enhanced this index, creating the ‘modified Topographic Index’ (TIm), which, when coupled with hydraulic simulations, demonstrated its ability to identify areas at risk of flooding. This breakthrough paved the way for various applications utilizing geomorphic indices like TI and TIm for flood-prone area delineation (e.g., Degiorgis et al., 2012; Suwandana et al. 2012; Knitter et al., 2012; Jalayer et al., 2014; Cooper, 2014; Papaioannou et al., 2015; De Risi et al., 2014; Pourali et al., 2016). Researchers have either employed the original procedure by Manfreda et al. (2011) and the associated GRASS GIS tool (Di Leo et al., 2011) or adapted it with new calibration strategies (e.g., Jalayer et al., 2014).
Beyond the modified topographic index, researchers have explored the potential of geomorphological features for flood-prone area delineation. These methods have evolved from basic basin information to highly accurate techniques over time.
Notably, Degiorgis et al. (2012) proposed a method to extrapolate flood-prone areas from locations with flood maps to areas without them. This involved a threshold binary classification to identify the optimal threshold (TH) of a selected flood descriptor, derived from DEMs, correlating with flood susceptibility.
Building upon these foundations, Manfreda et al. (2014, 2015) and Samela et al. (2016) improved upon Degiorgis et al.’s (2012) method by evaluating different descriptors for their effectiveness in delineating flood-prone areas. Among these, the Geomorphic Flood Index (GFI) emerged as the top-performing and most consistent hydrogeomorphic descriptor (Manfreda et al., 2015; Samela et al., 2016, 2017), alongside the Height Above the Nearest Drainage (HAND). This research opened up the possibility of delineating flood-prone areas on a continental scale, drastically reducing computational time and costs (Samela et al., 2017; Tavares da Costa et al., 2019a). In Tavares da Costa et al. (2019a), optimal GFI thresholds were shown to correlate with specific flood return periods.
The intrinsic properties of the GFI have been further investigated by Manfreda and Samela (2019) to obtain an approximate, but immediate estimate of the water surface elevation in a river and surrounding areas. Surface elevations can be coupled with the vulnerability of exposed assets to obtain an assessment of the direct economic damage in a simple and effective way (Albano et al., 2020).
Recent advancements have also taken place. Balestra et al. (2022) introduced a rapid methodology that employs machine learning techniques to delineate flood-prone areas. By combining historical flood data and globally applicable conditioning factors, this approach offers quick flood susceptibility analysis, particularly in regions lacking flood hazard maps.
Explore the wealth of knowledge we’ve compiled on flood risk assessment and management, from historical research to cutting-edge machine learning solutions. Together, we can better understand and address the challenges posed by floods.
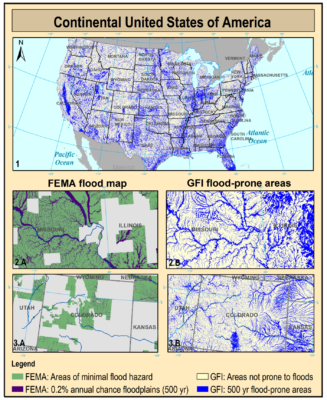
Reference
Albertini, C., A. Gioia, V. Iacobellis, G. P. Petropoulos and S. Manfreda, Assessing Multi-source Random Forest Classification and Robustness of Predictor Variables in Flooded Areas Mapping, Remote Sensing Applications: Society and Environment, (doi: 10.1016/j.rsase.2024.101239) 2024. [pdf]
Albertini, C., A. Gioia, V. Iacobellis, S. Manfreda, Detection of Surface Water and Floods with Multispectral Satellites. Remote Sensing, 14, 6005, (doi: 10.3390/rs14236005), 2022. [pdf]
Balestra, F.; Del Vecchio, M.; Pirone, D.; Pedone, M.A.; Spina, D.; Manfreda, S.; Menduni, G.; Bignami, D.F. Flood Susceptibility Mapping Using a Deep Neural Network Model: The Case Study of Southern Italy. Environ. Sci. Proc.2022, 21, 36. [pdf]
Albertini, C., D. Miglino, V. Iacobellis, F. De Paola, S. Manfreda, Flood-prone areas delineation in coastal regions using the Geomorphic Flood Index, Journal of Flood Risk Management, e12766, 2021. [pdf]
Albano, R., Samela, C., Crăciun, I., Manfreda, S., Adamowski, J., Sole, A., … & Ozunu, A. Large Scale Flood Risk Mapping in Data Scarce Environments: An Application for Romania. Water, 12(6), 1834, 2020. [pdf]
Tavares da Costa, R., S. Zanardo, S. Bagli, A. G. J. Hilberts, S. Manfreda, C. Samela, and A. Castellarin, Predictive modelling of envelope flood extents using geomorphic and climatic-hydrologic catchment characteristics, Water Resources Research, 2020. [pdf]
Tavares da Costa, R., S. Manfreda, V. Luzzi, C. Samela, P. Mazzoli, A. Castellarin, S. Bagli, A web application for hydrogeomorphic flood hazard mapping, Environmental Modelling and Software, 2019. [pdf]
Manfreda, S., C. Samela, A DEM-based method for a rapid estimation of flood inundation depth, Journal of Flood Risk Management, 12 (Suppl. 1):e12541, (doi: 10.1111/jfr3.12541) 2019. [pdf]
Manfreda S., C. Samela, A. Refice, V. Tramutoli, F. Nardi, Advances in Large Scale Flood Monitoring and Detection, Hydrology, 5, 49, 2018. [pdf]
Samela, C., R. Albano, A. Sole, S. Manfreda, A GIS tool for cost-effective delineation of flood-prone areas, Computers, Environment and Urban Systems, 70, 43-52, 2018. [pdf]
Samela, C., T.J. Troy, S. Manfreda, Geomorphic classifiers for flood-prone areas delineation for data-scarce environments, Advances in Water Resources, 102, 13-28, 2017. [pdf]
Samela C., S. Manfreda, T. J. Troy, 100-year geomorphic flood-prone areas for the continental U.S., Data in Brief, 12, 203-207, 2017. [pdf]
D’Addabbo, A., A. Refice, G. Pasquariello, F. Lovergine, D. Capolongo and S. Manfreda, A Bayesian Network for Flood Detection Combining SAR Imagery and Ancillary Data, IEEE Transactions on Geoscience and Remote Sensing, 54(6), 3612 -3625, 2016. [pdf]
Samela, C., S. Manfreda, F. De Paola, M. Giugni, A. Sole, M. Fiorentino, DEM-based approaches for the delineation of flood prone areas in an ungauged basin in Africa, Journal of Hydrologic Engineering, 21(2), 2016. [pdf]
Manfreda, S., C. Samela, A. Gioia, G. Consoli, V. Iacobellis, L. Giuzio, A. Cantisani, A. Sole, Flood-Prone Areas Assessment Using Linear Binary Classifiers based on flood maps obtained from 1D and 2D hydraulic models, Natural Hazards, 79 (2), 735-754, 2015. [pdf]
Manfreda, S., F. Nardi, C. Samela, S. Grimaldi, A. C. Taramasso, G. Roth and A. Sole, Investigation on the Use of Geomorphic Approaches for the Delineation of Flood Prone Areas, Journal of Hydrology, 517, 863-876, 2014. [pdf]
Manfreda, S. and Sole, A. Closure to “Detection of Flood-Prone Areas Using Digital Elevation Models” by Salvatore Manfreda, Margherita Di Leo, and Aurelia Sole. Journal of Hydrologic Engineering, 18(3), 362-365, 2013. [pdf]
Manfreda, S., M. Di Leo, A. Sole, Detection of Flood Prone Areas using Digital Elevation Models, Journal of Hydrologic Engineering, 16(10), 781-790, 2011. [pdf]